Gaussian Synapses for Analog Computation
ID# 2019-4942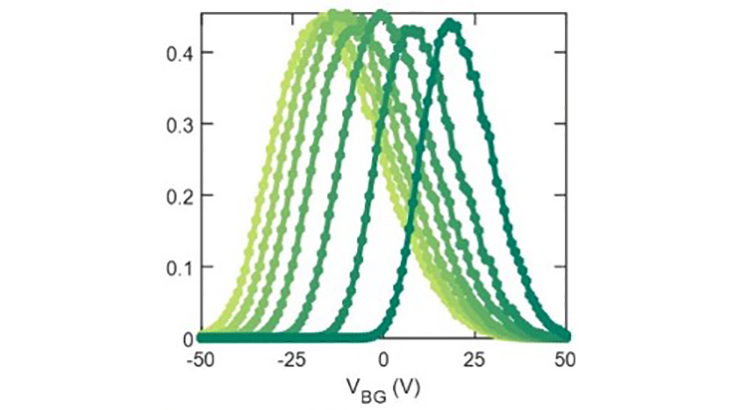
Technology Summary
Computing technology has evolved to encompass two basic genres: high-performance computing systems, utilizing the fastest and most powerful hardware to achieve a deterministic solution, and probabilistic computing, where AI, deep learning, probabilistic models, etc., can be solved for by making approximations. This technology enables a hardware implementation of Probabilistic Neural Networks (PNN) that can be used for pattern recognition and classification, among other applications. As the market grows for these applications, so do the resource requirements to solve ever more complex problems and larger datasets. The research team has developed Gaussian synapses on relatively simple 2D semiconductor components, enabling the PNNs but with power requirements 3 to 6 orders of magnitude less than typical implementations.
Application & Market Utility
This tech offers a triad of advantages: size-scaling through the use of 2D materials, energy scaling via Gaussian synapses (GS), and complexity scaling by enabling the PNN. GS are inherently low power since they exploit the subthreshold FET characteristics, making their energy requirements 3 to 6 orders of magnitude less than competing implementations. By using only two transistors and thin 2D layered semiconductors, area and energy efficiency at the device level are significantly improved and provides cascading benefits at the circuit, architecture, and system levels.
Next Steps
This technology is patent pending. The research team seeks collaboration for further development and licensing opportunities.