Genomic Word Frameworks of Methylation Motifs for Predictive Modeling
ID# 2022-5368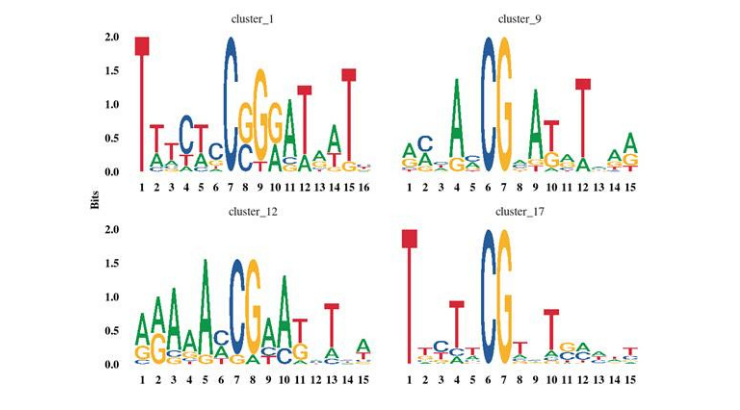
Technology Summary
DNA methylation motifs identify potential epigenetic regulatory functionalities. The invention comprises a computational heuristic to identify the potential genomic-word-framework (GWF) regions. The inventors employed a programming language for methylation analyses and GWF analyses that implements a signal detection and a machine-learning approach permitting the filtering of signal from noise at a high rate. Results indicated that their GWF-based heuristics allows one to identify DNA sequences of methylation motifs with high order DNA base interdependence with respect to methylated cytosines and a base distribution that is statistically nonrandom. Differentially methylated genes (DMGs) identified with methylation analysis may be integrated to gene networks to identify network hubs via protein-protein interactions network analysis and weighted correlation network analyses.
Application & Market Utility
The inventors validated the invention in Arabidopsis, tomato and soybean, before demonstrating broader utility with a DNA methylation analysis of placental tissues of typically developing and autistic children. Said models may discover epigenetic causes for not well-understood phenotypes and multifactorial diseases seemingly caused by both genetic and environmental factors, such as drought and cold tolerance in plants as well as cancer, diabetes, obesity and alcoholism in humans and other animals that would benefit from early detection and treatment monitoring.
Next Steps
Using identified differentially methylated genes, one can build methylation motif libraries that serve as the fundamental dataset needed to build predictive machine-learning models with applications in plant science and biomedical research.