Optimal Sequential Decision Making Under Partial Observability Using Deep Reinforcement Learning
ID# 2021-5355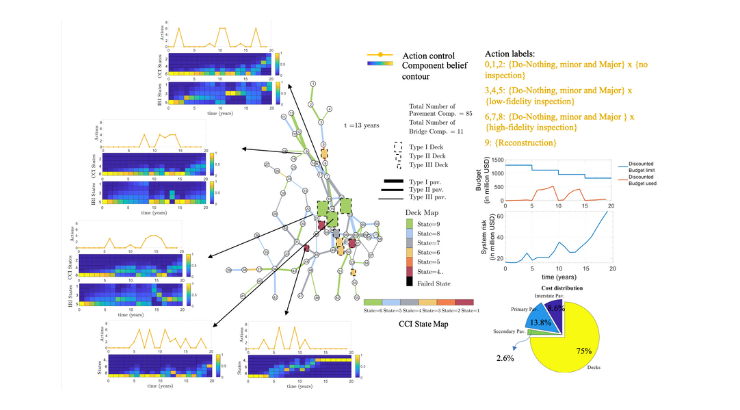
Technology Summary
The disclosed technology presents a system and method described for optimal sequential decision-making for large engineering systems/networks with multiple components under uncertain and partially observed environments using multi-agent deep reinforcement learning architecture. The technology uses a partially observable Markov decision process with a confluence of state-of-the-art AI methodology, named Deep Decentralized Multi-Agent Actor-Critic (DDMAC) with Centralized Training and Decentralized Execution (CTDE), that can incorporate operation-related various resource, performance, and risk-related constraints.
Application & Market Utility
The current solutions for managing large infrastructure systems are based on heuristics and usually suffer from inefficiencies arising from complex systems. To address these issues, a comprehensive artificial intelligence framework was created that allows for adaptive evaluation in the presence of noisy real-time or ambiguous data. This technology was tested on an existing transportation network in Virginia, where results show a total of 45% cost savings in managing the network and an improvement of 20% over heuristically optimized condition-based policy. This technology has the potential to save up to billions of dollars upon implementation in the energy, aerospace, and automotive industries.
Next Steps
The research team is continually testing the technology and seeking licensing opportunities.